Numpy เบื้องต้น - 1D
Contents
Numpy เบื้องต้น - 1D#
30 minutes
วัตถุประสงค์
หลังจากทำทำแล็บ นศ.จะสามารถ
Import ไลบรารี่ (Library)
numpy
และใช้งานไลบรารี่เบื้องต้นได้ดำเนินการทางคณิตศาสตร์กับข้อมูล
NumPy arrays
(อาร์เรย์ 1 มิติ) ได้Import ไลบรารี่ (Library)
matplotlib
และพล็อตกราฟอย่างง่ายได้
Ref:
import
ไลบรารี่ NumPy#
หนึ่งในจุดเด่นของภาษาไพธอนคือ มีไลบรารีเสริมมากมายครอบคลุมการใช้งานที่หลากหลาย
ในบรรดาไลบรารีต่างๆ numpy และ matplotlib เป็น 2 ไลบรารีที่มีความสำคัญและใช้กันอย่างแพร่หลายที่สุด
เรามักจะใช้ numpy คำนวณและวิเคราะห์ข้อมูลเชิงตัวเลข จากนั้นนำข้อมูลออกมาแสดงผลเป็นกราฟและแผนภาพต่างๆ ให้เห็นภาพชัดเจนยิ่งขึ้นโดยใช้ matplotlib
(หากใช้ไลบรารี numpy + matplotlib + scipy จะทำให้ไพธอนทัดเทียมกับโปรแกรม matlab (แมตแล็บ) โดยที่ไม่ต้องเสียค่าใช้จ่าย และเมื่อใช้ร่วมกับไลบรารี pandas + scikit-learn + ฯลฯ ก็จะทำให้ไพธอนเหนือกว่า matlab ขึ้นไปอีก)
แบบที่ 1:
import <library_name> as <alias_name>
แบบที่ 2:
import <library_name>
แบบที่ 3:
from <library_name> import <variable(sub_module/sub_package/method)>
from <library_name> import <variable(sub_module/sub_package/method)> as <alias_name>
แบบที่ 4:
from <library_name> import *
# Import the libraries
import time
import sys
import numpy as np # To define an alias for an imported module
# import the pyplot module of the matplotlib library under the alias plt.
import matplotlib.pyplot as plt
%matplotlib inline
# Plotting functions using matplotlib
def Plotvec1(u, z, v):
ax = plt.axes() # Creating a new full window axes
ax.arrow(0, 0, *u, head_width=0.1, color='r', head_length=0.1) # Axes.arrow(x, y, dx, dy, **kwargs)[source] : This draws an arrow from (x, y) to (x+dx, y+dy)
plt.text(*(u + 0.1), 'u') # matplotlib.pyplot.text(x, y, s, fontdict=None, **kwargs)[source] : Add the text s to the Axes at location x, y in data coordinates.
ax.arrow(0, 0, *v, head_width=0.1, color='b', head_length=0.1)
plt.text(*(v + 0.1), 'v')
ax.arrow(0, 0, *z, head_width=0.1, head_length=0.1)
plt.text(*(z + 0.1), 'z')
#plt.ylim(-2, 2) # Fixed Y-scale
#plt.xlim(-2, 2) # Fixed X-scale
ax.autoscale() # Auto-scale
ax.set_aspect('equal')
def Plotvec2(a,b):
ax = plt.axes() # Creating a new full window axes
ax.arrow(0, 0, *a, head_width=0.1, color ='r', head_length=0.1)
plt.text(*(a + 0.1), 'a')
ax.arrow(0, 0, *b, head_width=0.1, color ='b', head_length=0.1)
plt.text(*(b + 0.1), 'b')
plt.ylim(-2, 2)
#plt.ylim(-2, 2)
#plt.xlim(-2, 2)
ax.autoscale() # Auto-scale
ax.set_aspect('equal')
สร้างลิสต์ (List) ขึ้นมา
# Create a python list using square brackets.
a = ["0", 1, "two", "3", 4]
เราสามารถเข้าถึงสมาชิกภายในได้โดยใช้ index
เข้าถึงสมาชิกแต่ละตัวในลิสต์โดยใช้เครื่องหมายวงเล็บเหลี่ยม [ ] (square brackets) ดังนี้
# Print each element
print("a[0]:", a[0])
print("a[1]:", a[1])
print("a[2]:", a[2])
print("a[3]:", a[3])
print("a[4]:", a[4])
a[0]: 0
a[1]: 1
a[2]: two
a[3]: 3
a[4]: 4
ลิสต์ไม่ได้ถูกทำมาเพื่อใช้ในการคำนวณโดยเฉพาะ ทำให้ไม่สามารถคำนวณได้โดยตรง หากเราต้องการจะนำอาร์เรย์มาบวกลบหรือทำอะไรก็ตาม จะพบว่าผลลัพธ์ไม่เป็นไปอย่างที่ต้องการ
เราลองสร้าง List ขึ้นมาสองตัวแปร x
และ y
x = [0, 1, 2, 3, 4]
y = [9, 8, 7, 6, 5]
print(x+y)
[0, 1, 2, 3, 4, 9, 8, 7, 6, 5]
ผลที่ได้ไม่ใช่การนำอาร์เรย์มาบวกกัน ซึ่งผลควรจะได้เป็น [9, 9, 9, 9, 9] แต่กลับกลายเป็นการเอามาต่อกัน
หากต้องการให้บวกเมทริกซ์จะต้องทำการวนซ้ำเพื่อให้มีการคำนวณที่ตัวส่วนประกอบแต่ละตัว
#z = [0, 0, 0, 0, 0]
z = [0 for i in x]
for i in range(len(x)):
z[i] = x[i]+y[i]
print(z)
[9, 9, 9, 9, 9]
จะเห็นว่ามีความยุ่งยาก หากสามารถทำให้เขียนแค่ x+y แล้วบวกกันได้เลย ชีวิตก็คงจะง่ายขึ้น!
สิ่งที่จะตอบโจทย์นี้ได้ก็คือ numpy นั่นเอง
ถ้า x และ y ไม่ใช่ลิสต์แต่เป็นออบเจ็กต์ ndarray ของ numpy แล้วละก็ x+y ก็จะเป็นการบวกอาร์เรย์ทันที นี่คือความสะดวกของ numpy
แต่ไม่ใช่แค่นั้น นอกจากจะทำให้เขียนโค้ดสะดวกขึ้นแล้ว ยังทำให้การคำนวณเร็วขึ้นมากด้วย
ไพธอนเป็นภาษาที่ถูกออกแบบมาเพื่อให้เขียนง่ายต่อการเขียนและมีความยืดหยุ่นสูง แต่ก็มีข้อเสียคือทำงานช้าเมื่อเทียบกับภาษาระดับที่สูงไม่มากอย่างภาษา C หรือ Fortan
numpy เป็นไลบรารีที่มีเบื้องหลังการทำงานเป็นภาษาซี ดังนั้นจึงมีความเร็วสูงในการคำนวณมากกว่า
NumPy คืออะไร?#
NumPy (Numerical Python) เป็นไลบรารีที่สำคัญมากที่สุดในไพธอนเลยก็ว่าได้ เนื่องจากใช้สำหรับการคำนวณทางคณิตศาสตร์ที่ซับซ้อนได้อย่างมีประสิทธิภาพ เช่นการเขียนชุดตัวเลขให้อยู่ในรูปแมทริกซ์ ซึ่งทำให้สะดวกต่อการเขียนโค้ดและการคำนวณอย่างมากมาก
NumPy มีออบเจ็กต์พิเศษชนิดหนึ่งที่ชื่อว่า ndarray (N-d Array; N-dimensional array (multidimensional, homogeneous array)) ซึ่งเป็นออบเจ็กต์ที่เก็บข้อมูลเป็นกลุ่มเป็นแถว สามารถเก็บเป็นหลายมิติได้และสามารถนำข้อมูลภายในมาคำนวณได้อย่างรวดเร็ว
อาร์เรย์ (Arrays) เป็นคุณสมบัติหลักของ NumPy NumPy Arrays มีลักษณะคล้ายกับ List (เก็บข้อมูลเป็นชุดของข้อมูลที่มีลำดับของข้อมูล) ยกเว้น สมาชิกทุกตัวในอาร์เรย์จะต้องเป็นข้อมูลชนิดเดียวกัน โดยทั่วไปแล้วข้อมูลที่เก็บในอาร์เรย์จะเป็นตัวเลขเช่น int หรือ float
เนื่องจาก NumPy เป็นไลบรารี่ 3rd-party จึงต้องติดตั้งก่อนใช้งาน และตอนเขียนโค้ดต้อง import
ไลบรารี่ก่อน
# Let’s install the Numpy library
# !pip install numpy
# import numpy library
import numpy as np
เวลาที่เรียกชื่อฟังก์ชันต่างๆใน Numpy จะเรียกโดยขึ้นต้นด้วย np.
Module (โมดูล) คือ Python File (.py files) ที่เก็บฟังก์ชั่น คลาสต่างๆ และตัวแปรโกบอลที่ใช้เป็นตัวชี้บ่งสถานะ (Flag). ตัวอย่างของโมดูล เช่น math
Package (แพ็คเกจ) ก็คือ โฟลเดอร์ (Folder/Directory) ที่เก็บไพธอนโมดูลต่างๆ โดยภายในแพ็คเกจจะมีไฟล์ init.py กำหนดไว้ในโฟลเดอร์ เพื่อบ่งบอกว่าเป็นโฟลเดอร์ของแพ็คเกจ (ไฟล์นี้สามารถเป็นไฟล์ว่าง (Empty file) ได้) ตัวอย่างของแพ็คเกจ เช่น numpy, Requests, Matplotlib
Library (ไลบรารี) คือ แพ็คเกจต่างๆ ที่ถูกรวบรวมไว้ โดยทั่วไปแล้ว ไพธอนแพ็คเกจกับไพธอนไลบรารีไม่ต่างกัน
การสร้างชุดข้อมูลอาร์เรย์ (1D Numpy Array)#
การสร้างอาเรย์มีอยู่หลายวิธี แต่วิธีที่พื้นฐานที่สุดคือสร้างขึ้นมาจาก List, Tuple หรือ Range โดยใช้ np.array
เราลองเปลี่ยนชุดข้อมูล List ข้างต้นให้เป็นอาร์เรย์
help(np.array)
Help on built-in function array in module numpy:
array(...)
array(object, dtype=None, *, copy=True, order='K', subok=False, ndmin=0,
like=None)
Create an array.
Parameters
----------
object : array_like
An array, any object exposing the array interface, an object whose
__array__ method returns an array, or any (nested) sequence.
If object is a scalar, a 0-dimensional array containing object is
returned.
dtype : data-type, optional
The desired data-type for the array. If not given, then the type will
be determined as the minimum type required to hold the objects in the
sequence.
copy : bool, optional
If true (default), then the object is copied. Otherwise, a copy will
only be made if __array__ returns a copy, if obj is a nested sequence,
or if a copy is needed to satisfy any of the other requirements
(`dtype`, `order`, etc.).
order : {'K', 'A', 'C', 'F'}, optional
Specify the memory layout of the array. If object is not an array, the
newly created array will be in C order (row major) unless 'F' is
specified, in which case it will be in Fortran order (column major).
If object is an array the following holds.
===== ========= ===================================================
order no copy copy=True
===== ========= ===================================================
'K' unchanged F & C order preserved, otherwise most similar order
'A' unchanged F order if input is F and not C, otherwise C order
'C' C order C order
'F' F order F order
===== ========= ===================================================
When ``copy=False`` and a copy is made for other reasons, the result is
the same as if ``copy=True``, with some exceptions for 'A', see the
Notes section. The default order is 'K'.
subok : bool, optional
If True, then sub-classes will be passed-through, otherwise
the returned array will be forced to be a base-class array (default).
ndmin : int, optional
Specifies the minimum number of dimensions that the resulting
array should have. Ones will be prepended to the shape as
needed to meet this requirement.
like : array_like, optional
Reference object to allow the creation of arrays which are not
NumPy arrays. If an array-like passed in as ``like`` supports
the ``__array_function__`` protocol, the result will be defined
by it. In this case, it ensures the creation of an array object
compatible with that passed in via this argument.
.. versionadded:: 1.20.0
Returns
-------
out : ndarray
An array object satisfying the specified requirements.
See Also
--------
empty_like : Return an empty array with shape and type of input.
ones_like : Return an array of ones with shape and type of input.
zeros_like : Return an array of zeros with shape and type of input.
full_like : Return a new array with shape of input filled with value.
empty : Return a new uninitialized array.
ones : Return a new array setting values to one.
zeros : Return a new array setting values to zero.
full : Return a new array of given shape filled with value.
Notes
-----
When order is 'A' and `object` is an array in neither 'C' nor 'F' order,
and a copy is forced by a change in dtype, then the order of the result is
not necessarily 'C' as expected. This is likely a bug.
Examples
--------
>>> np.array([1, 2, 3])
array([1, 2, 3])
Upcasting:
>>> np.array([1, 2, 3.0])
array([ 1., 2., 3.])
More than one dimension:
>>> np.array([[1, 2], [3, 4]])
array([[1, 2],
[3, 4]])
Minimum dimensions 2:
>>> np.array([1, 2, 3], ndmin=2)
array([[1, 2, 3]])
Type provided:
>>> np.array([1, 2, 3], dtype=complex)
array([ 1.+0.j, 2.+0.j, 3.+0.j])
Data-type consisting of more than one element:
>>> x = np.array([(1,2),(3,4)],dtype=[('a','<i4'),('b','<i4')])
>>> x['a']
array([1, 3])
Creating an array from sub-classes:
>>> np.array(np.mat('1 2; 3 4'))
array([[1, 2],
[3, 4]])
>>> np.array(np.mat('1 2; 3 4'), subok=True)
matrix([[1, 2],
[3, 4]])
Syntax ของการสร้างชุดข้อมูลอาร์เรย์ (nampy.array Syntax)
np.array(object, dtype=None)
# Create a numpy array (integer array)
np.array([0, 1, 2, 3, 4])
array([0, 1, 2, 3, 4])
# Create a numpy array
a = np.array([0, 1, 2, 3, 4])
a
array([0, 1, 2, 3, 4])
สมาชิกแต่ละตัวในอาร์เรย์ต้องเป็นข้อมูลชนิดเดียวกัน ในกรณีคือ int
เช่นเดียวกับ List เราสามารถเข้าถึงข้อมูลภายในโดยอ้างถึงหมายเลขดัชนี (index) ผ่านเครื่องหมายวงเล็บเหลี่ยม [ ] (square brackets)
# Print each element
print("a[0]:", a[0])
print("a[1]:", a[1])
print("a[2]:", a[2])
print("a[3]:", a[3])
print("a[4]:", a[4])
a[0]: 0
a[1]: 1
a[2]: 2
a[3]: 3
a[4]: 4
เราสามารถเข้าถึงสมาชิกทีละหลายๆ ตัวได้ด้วยการใช้โคลอน : เช่นเดียวกับลิสต์
print(a[0:3])
[0 1 2]
หากมี : เพิ่มมาอีกตัว แล้ววางตัวเลขไว้ทางขวาของ : จะเป็นการกำหนดระยะเว้นช่วง (เหมือน List)
print(a[::2])
[0 2 4]
และหากใส่เลขติดลบ ก็จะกลายเป็นการไล่ถอยหลัง (เหมือน List)
print(a[::-2])
[4 2 0]
จะเห็นว่า หากต้องการกลับลำดับสมาชิกในอาเรย์ทั้งหมด (เรียงจากหลังไปหน้า) ก็แค่ใส่ [::-1] ไปเท่านั้น เป็นการใช้งานที่สะดวกมาก
# Reverse
print(a[::-1])
[4 3 2 1 0]
np.array([1, 4, 2, 5, 3])
array([1, 4, 2, 5, 3])
เราสามารถสร้างอาเรย์โดยใช้ List Comprehension ได้ เช่น
# nested lists result in 1-dimensional array
np.array([i*2 for i in range(10)])
array([ 0, 2, 4, 6, 8, 10, 12, 14, 16, 18])
a = np.array([i*i for i in range(50) if i%2!=1])
a
array([ 0, 4, 16, 36, 64, 100, 144, 196, 256, 324, 400,
484, 576, 676, 784, 900, 1024, 1156, 1296, 1444, 1600, 1764,
1936, 2116, 2304])
NumPy arange() เป็นอีกวิธีหนึ่งในการสร้าง array (เรียกว่า NumPy routines สำหรับสร้าง array) โดยมี
Syntax:
numpy.arange(start, stop, step, dtype)
โดย dtype คือ ชนิดข้อมูลของสมาชิกใน array หากไม่ระบุจะประเมินให้อัตโนมัติโดยพิจารณาจากชนิดข้อมูล start stop และ step
# Create an array filled with a linear sequence
# Starting at 0, ending at 20, stepping by 2
# (this is similar to the built-in range() function)
np.arange(0, 20, 2)
array([ 0, 2, 4, 6, 8, 10, 12, 14, 16, 18])
# Boolean Indexing
# We can also set conditionals inside square brackets to filter elements out.
q = np.arange(0, 20, 2)
q[q<10]
array([0, 2, 4, 6, 8])
สิ่งที่แตกต่างระหว่าง NumPy arange() กับฟังก์ชั่น range() อีกอย่างก็คือ NumPy arange() สามารถเป็นเลขชุดทศนิยมได้ ในขณะที่ range() เป็นเฉพาะจำนวนเต็มเท่านั้น
for i in np.arange(0, 10, 2.5):
print(i)
0.0
2.5
5.0
7.5
for i in range(0,10, 2):
print(i)
0
2
4
6
8
ชนิดของข้อมูลในอาร์เรย์#
ถ้าเราตรวจสอบชนิดของข้อมูล จะพบว่าเป็นชนิด (ออบเจ็กต์) numpy.ndarray (N-d Array; N-dimensional array (multidimensional, homogeneous array))
# Check the type of the array
type(a)
numpy.ndarray
สมาชิกทุกตัวในอาร์เรย์ต้องเป็นข้อมูลชนิดเดียวกัน เราสามารถตรวจสอบชนิดของข้อมูลที่เก็บในอาร์เรย์ได้โดยเรียกแอตทริบิวต์ (attribute) dtype
ในกรณีนี้เป็นชนิดจำนวนเต็ม 64-bit)
# Check the type of the values stored in numpy array
a.dtype
dtype('int64')
ตอนสร้างสร้างอาร์เรย์ หากมีสมาชิกที่มีทศนิยมแม้แต่ตัวเดียวทั้งหมดก็จะกลายเป็น float64 ทันที
# Create a numpy array with real numbers
b = np.array([0, 1, 2, 3, 4, 3.14])
b
array([0. , 1. , 2. , 3. , 4. , 3.14])
# Create a numpy array with real numbers
b = np.array([0, 1, 2, 3, 4, 3.14159265359])
b
array([0. , 1. , 2. , 3. , 4. ,
3.14159265])
จะเห็นว่าข้อมูลในอาเรย์จะต้องเป็นข้อมูลที่มีชนิดเดียวกันและมีขนาดเท่ากันหมดทุกตัว หากตอนที่สร้างอาเรย์มีสมาชิกที่ชนิดต่างกันจะถูกทำให้เหมือนกันหมด!
ถ้าเราตรวจสอบชนิดของข้อมูล จะพบว่าเป็นชนิด numpy.ndarray
# Check the type of array
type(b)
numpy.ndarray
เราสามารถตรวจสอบชนิดของข้อมูลโดยเรียกแอตทริบิวต์ (attribute) dtype
ในกรณีนี้เป็นชนิด float 64
ไม่ใช่จำนวนเต็ม
# Check the value type
b.dtype
dtype('float64')
การกำหนดค่าใหม่ให้กับอาร์เรย์ (เขียนทับข้อมูล)#
เช่นเดียวกับ List เราสามารถเปลี่ยนค่าอาร์เรย์เมื่อไหร่ก็ได้โดยการใส่ค่าใหม่ทับลงไป
ตัวอย่าง เราสร้างอาร์เรย์ c
ขึ้นมาก่อนโดยใช้ลิสต์
# Create numpy array
c = np.array([20, 1, 2, 3, 4])
c
array([20, 1, 2, 3, 4])
เราสามารถเปลี่ยนค่าของสมาชิกตัวแรกให้เป็น 100
# Assign the first element to 100
c[0] = 100
c
array([100, 1, 2, 3, 4])
เราสามารถเปลี่ยนสมาชิกตัวสุดท้ายให้มีค่าเป็น 0 ได้โดยใช้ดัชนีเชิงลบ (Negative indexing) เหมือนกับ List
# Assign the last element to 0
c[-1] = 100
c
array([100, 1, 2, 3, 100])
เราสามารถกำหนดค่าให้กับสมาชิกมากกว่าหนึ่งตัวได้โดยระบุช่วงของข้อมูล ดังนี้ (ทำได้เหมือนกับ List)
# Set the fourth element and fifth element to 300 and 400
c[3:5] = 300, 400
c
array([100, 1, 2, 300, 400])
หากต้องการเปลี่ยนค่าของสมาชิกทุกตัวให้เป็น 100 ก็สามารถทำได้ (List ใชรูปแบบคำสั่งแบบนี้ทำไม่ได้)
c[:] = 100
c
array([100, 100, 100, 100, 100])
การกำหนดค่าด้วยลิสต์ดัชนี (Integer array indexing)#
# Recreate numpy array
c = np.array([0, 1, 2, 3, 4])
c
array([0, 1, 2, 3, 4])
เราสามารถใช้ลิสต์เป็นดัชนีเพื่อกำหนดเฉพาะดัชนีที่ต้องการได้
เราจะสร้างลิสต์ select
ขึ้นมา ซึ่งภายในมีเลขดัชนีหลายค่า
# Create the index list
select = [0, 2, 4]
เราสามารถใช้ลิสต์ดัชนีเป็นอาร์กิวเมนต์ในวงเล็บสี่เหลี่ยมได้
# Use List to select elements
d = c[select]
d
array([0, 2, 4])
c[[0, 2, 4]]
array([0, 2, 4])
นอกจากนี้ยังสามารถใช้ลิสต์ดัชนีแก้ไขค่าของสมาชิกได้อีกด้วย ยกตัวอย่างเช่น เปลี่ยนค่าเป็น 100000
# Assign the specified elements to new value
c[select] = 100000
c
array([100000, 1, 100000, 3, 100000])
# c[0, 2, 4] = 0
c[[0, 2, 4]] = 0
c
array([0, 1, 0, 3, 0])
แอตทริบิวต์และเมธอดอื่นๆ ของอาร์เรย์#
นอกจากแอตทริบิวต์ dtype
แล้ว ยังมีแอตทริบิวต์อื่นๆ อีกที่สามารถให้ข้อมูลของตัวอาเรย์นั้นๆ ได้ เช่น
size
จำนวนสมาชิกในอาเรย์, ndim
จำนวนมิติของอาเรย์, shape
รูปร่างของอาเรย์
เราจะเรียนรู้แอตทริบิวต์อื่นๆ ของอาร์เรย์โดยผ่านอาร์เรย์ a
ก่อนอื่น สร้างอาร์เรย์ a
เลขจำนวนเต็ม จำนวน 100 ตัวเลข โดยการเรียกใช้ฟังก์ชั่น randint()
ฟังก์ชั่น randint() ใช้สุ่ม (Random) ตัวเลขจำนวนเต็ม โดยจะทำการสุ่มจำนวนเต็มที่อยู่ในช่วงที่กำหนด (low - high) ออกมาตามจำนวน size ที่กำหนด
numpy.random.randint(low, high=None, size=None, dtype=int)
a = np.random.randint(-100,100,100)
a
array([-96, -50, 69, 43, 14, -61, -86, -47, -7, -19, 37, -76, -53,
86, 76, 60, 71, 47, 99, -47, 62, 11, 12, -4, 45, -73,
10, -78, 91, -59, 78, 25, -39, -50, -82, -21, -65, -79, 29,
61, 54, -59, -45, -68, 25, -22, 66, 59, 51, -83, -49, 52,
49, -21, 20, 89, -3, 19, 6, 82, -80, -86, 73, -7, 60,
-77, 78, -77, -17, 93, 89, 70, -19, 4, 3, 72, 28, -42,
-96, -74, 23, 8, -61, 26, 72, 95, -47, 34, 9, -75, 53,
26, -95, 31, -92, 65, 86, 18, -92, 50])
ดูข้อมูลกันก่อน โดยการพล็อตกราฟอย่างง่าย
plt.plot(a)
[<matplotlib.lines.Line2D at 0x11d1f9580>]
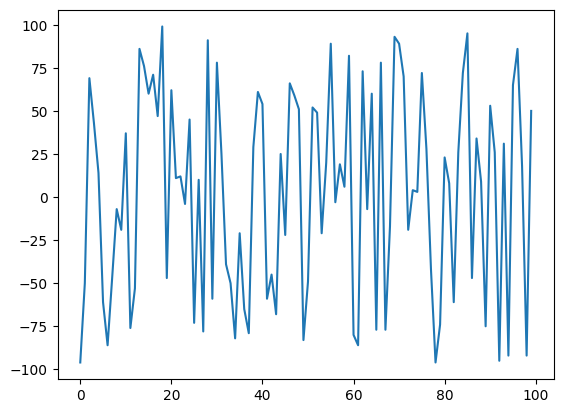
แอตทริบิวต์ size
จำนวนสมาชิกในอาร์เรย์
# Get the size of numpy array
a.size
100
ndim
(number of dimensions) จำนวนมิติของอาเรย์
# Get the number of dimensions of numpy array
a.ndim
1
shape
รูปร่างของอาเรย์ (จำนวนสามาชิกในแต่ละมิติ (dimension))
ตอนนี้อาร์เรย์ที่สร้างขึ้นเป็นแค่ 1 มิติ
# Get the shape/size of numpy array
a.shape
(100,)
แค่ถ้าเป็นอาร์เรย์ที่สร้างขึ้นเป็น 2 มิติ (2-dimension)
k = np.array([[1,2,3],
[4,5,6],
[7,8,9]
])
k.ndim
2
k.shape
(3, 3)
NumPy ยังมีเมธอด (Method) สำหรับการคำนวณค่าทางสถิติสำหรับชุดข้อมูลที่เก็บในอาร์เรย์ เช่น Mean, Max, Min, SD (Standard deviation) และ Var โดยเรียกด้วย mean(), max(), min(), std() และ var() ตามลำดับ เป็นต้น
ค่าเฉลี่ย (Mean) คือ ค่ากลางที่ได้จากการนำเอาข้อมูลแต่ละตัวมารวมกัน แล้วนำผลรวมที่ได้มาหารด้วยจำนวนข้อมูลทั้งหมด
ส่วนเบี่ยงเบนมาตรฐาน (SD; Standard Deviation) คือ ค่ารากที่สองของผลรวมของความแตกต่างระหว่างข้อมูลดิบกับค่าเฉลี่ยยกกำลังสอง (sum of squares ของผลต่าง) หารด้วยจำนวนข้อมูลทั้งหมด
ค่าความแปรปรวน (Variance) คือ ค่าที่คำนวณมาจาก ค่าเบี่ยงเบนมาตรฐาน หรือ ส่วนเบี่ยงเบนมาตรฐาน ยกกำลัง 2
ค่าสูงสุด (max) ค่าต่ำสุด (min)
# Get the biggest value in the numpy array
max_a = a.max()
max_a
99
# Get the smallest value in the numpy array
min_a = a.min()
min_a
-96
ค่าเฉลี่ย (Mean)
ถ้าหาแบบ Manual ค่าเฉลี่ย (Mean) จะสามารถหาได้จาก ผลรวมทั้งหมด/จำนวน
# Cal by manual
sum_a = a[0]+a[1]+a[2]+a[3]+a[4] # +... หมดแรงก่อน
sum_a
-20
sum_a = 0
for n in a:
sum_a += n
sum_a
285
ใช้คำสั่งที่สั้นกว่านั้น
sum_a = sum(a[:])
sum_a
285
แต่ถ้าใช้ Numpy
# numpy.ndarray.sum: Return the sum of the array elements over the given axis.
sum_a = a.sum()
sum_a
285
หาค่าเฉลี่ย (Mean) จาก ผลรวมทั้งหมด/จำนวน
# Cal by manual
mean_a = sum_a/a.shape[0]
mean_a
2.85
แต่ถ้าใช้ Numpy
# Get the mean of numpy array
mean_a = a.mean()
mean_a
2.85
ค่าเบี่ยงเบนมาตรฐาน (Standard deviation)
# Get the standard deviation of numpy array
std_a=a.std()
std_a
59.41874704165345
ค่าความแปรปรวน (Variance)
# Get the smallest value in the numpy array
var_a = a.var()
var_a
3530.5875000000005
นอกจากการเรียกใช้เมธอดแล้ว เรายังสามารถเรียกใช้งานในรูปแบบฟังก์ชันก็ได้ (ใช้ชื่อฟังก์ชั่นเหมือนกับชื่อเมธอด) เช่น
np.var(a)
3530.5875000000005
np.floor(b)
array([0., 1., 2., 3., 4., 3.])
ตัวดำเนินการสำหรับ Numpy Array#
เมื่อใช้ตัวดำเนินการทางคณิตศาสต์กับอาร์เรย์แล้ว จะได้ ndarray ชุดใหม่เสมอ
การบวกลบอาร์เรย์#
พิจารณา Numpy Array u
u = np.array([2, 1])
u
array([2, 1])
พิจารณา Numpy Array v
v = np.array([1, 2])
v
array([1, 2])
เราสามารถบวกอาร์เรย์ทั้งสองนี้แล้วกำหนดให้เป็นอาร์เรย์ z
# Numpy Array Addition
# To add two or more arrays use np.add(a,b) or the + sign.
#z = np.add(u, v)
z = u + v
z
array([3, 3])
การดำเนินข้างต้นเทียบเท่ากับการบวกเวกเตอร์
# Plot numpy arrays
Plotvec1(u, z, v)
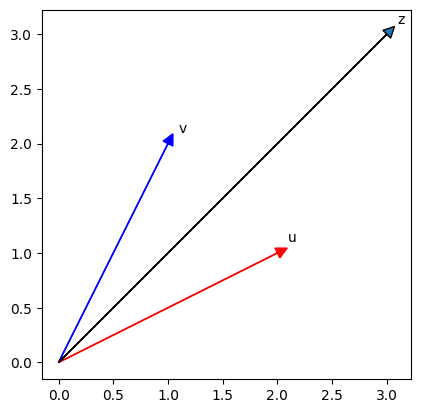
การลบก็เช่นเดียวกัน
# To subtract one array from another use the np.subtract(a,b) or the — sign
#z = np.subtract(u, v)
z = u - v
z
array([ 1, -1])
Plotvec1(u, z, v)
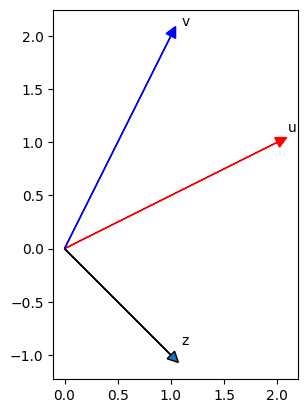
การคูณอาร์เรย์ (Multiplication) ด้วยสเกลาร์#
พิจารณา Numpy Array y
# Create a numpy array
y = np.array([1, 2])
y
array([1, 2])
เราสามารถคูณสมาชิกทุกตัวในอาร์เรย์ด้วย 2 ได้เลยโดยที่ไม่ต้องใช้คำสั่งลูป
# Numpy Array Multiplication
# To multiply array by scalar use np.multiply(a,b)or the * sign.
#z = np.multiply(2, y)
z = 2 * y
z
array([2, 4])
การดำเนินข้างต้นเทียบเท่ากับการคูณเวกเตอร์ด้วยสเกลาร์
Plotvec1(y, z, y)
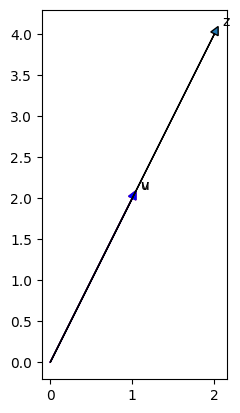
การคูณสองอาร์เรย์ (Product)#
พิจารณา Numpy Array u
# Create a numpy array
u = np.array([1, 2])
u
array([1, 2])
พิจารณา Numpy Array v
# Create a numpy array
v = np.array([3, 2])
v
array([3, 2])
การคูณอาร์เรย์สองอาร์เรย์ u
และ v
จะได้
# Calculate the production of two numpy arrays
# To multiply two arrays use np.multiply(a,b)or the * sign.
#z = np.multiply(u, v)
z = u * v
z
array([3, 4])
ซึ่งเป็นการคูณแต่ละสมาชิกที่อยู่ในตำแหน่งเดียวกันเป็นคู่ๆ (element-by-element)
นอกจากการคูณแล้ว การหาร การยกกำลัง และหารเอาเศษ ก็เป็นการคำนวณเป็นคู่ๆ ทีละตัว ดังตัวอย่างต่อไปนี้
# To divide two arrays usenp.divide(a,b)or the / sign.
print(u/v, '\n')
print(u**v, '\n')
print(u%v, '\n')
[0.33333333 1. ]
[1 4]
[1 0]
การคูณแบบ Dot Product#
ถ้าต้องการคูณสองอาร์เรย์แบบ Dot Product ระหว่าง u
และ v
ใช้ฟังก์ชั่น dot()
หรือใช้โอเปอร์เรเตอร์ @
# Calculate the dot product
u = np.array([1, 2])
v = np.array([3, 2])
np.dot(u, v)
7
u@v
7
การบวกและลบอาร์เรย์ด้วยค่าคงที่#
พิจารณา Numpy Array ต่อไปนี้
# Create a constant to numpy array
u = np.array([1, 2, 3, -1])
u
array([ 1, 2, 3, -1])
บวกค่าคงที่ 1 ให้กับแต่ละสมาชิกที่อยู่ในอาร์เรย์
# Add the constant to array
u + 1
array([2, 3, 4, 0])
สิ่งที่เกิดขึ้นสรุปเป็นรูปภาพได้ดังรูปต่อไปนี้
ฟังก์ชั่นทางคณิตศาสตร์#
ค่าของ \(\pi\) (Pi) ใน Numpy
# The value of pi
np.pi
3.141592653589793
เราสามารถสร้างอาร์เรย์ในหน่วยเรเดียน
# Create the numpy array in radians
x = np.array([0, np.pi/2 , np.pi])
x
array([0. , 1.57079633, 3.14159265])
เราสามารถใช้ฟังก์ชัน sin
กับอาร์เรย์ x
และให้ผลลัพท์เป็นอาร์เรย์ y
ซึ่งเป็นการหาค่าไซน์ของสมาชิกทุกตัวในอาร์เรย์ x
# Calculate the sin of each elements
y = np.sin(x)
y
array([0.0000000e+00, 1.0000000e+00, 1.2246468e-16])
# ใช้ฟังก์ชั่น np.radians()
x = np.array([0, np.radians(90) , np.radians(180)])
y = np.sin(x)
y
array([0.0000000e+00, 1.0000000e+00, 1.2246468e-16])
จากผลข้างต้น เราจพพบว่า sin(𝜋) ≠ 0 เนื่องจากไม่มีตัวเลขทศนิยมที่จะมาแทนค่า 𝜋 จริงๆ ได้ แก้ด้วยการประมาณค่าโดยใช้ฟังก์ชัน np.around()
np.around(y, decimals=5)
array([0., 1., 0.])
ค่าคงที่ที่สำคัญอื่นๆ ใน numpy
np.e
np.pi
np.nan
np.inf
นอกจากการคำนวณพื้นฐานแล้ว numpy ยังมีฟังก์ชันสำหรับคำนวณที่ใกล้เคียงกับในไลบรารี math
เช่น abs, sqrt, log, log10, exp, sin, cos, tan, arcsin, arccos, arctan, sinh, cosh, tanh, arcsinh, arccosh, arctanh
z = np.sqrt(x)
z
array([0. , 1.25331414, 1.77245385])
ฟังก์ชัน numpy.linspace (start, stop, n)#
ฟังก์ชั่นที่ใช้สร้างอาร์เรย์ใน NumPy อีกฟังก์ชั่น คือ ฟังก์ชั่น numpy.linspace()
ฟังก์ชั่นนี้คล้ายกับฟังก์ชั่น numpy.arange( )
แต่จะแทนที่จะระบุ step (ระยะห่างของข้อมูลแต่ละตัว) ฟังก์ชั่น numpy.linspace()
จะระบุเป็นจำนวนของข้อมูลที่ต้องการ โดยระยะห่างของข้อมูลแต่ละตัวจะถูกปรับให้มีขนาดเท่าๆ กันโดยอัตโนมัติ มักใช้กำหนดข้อมูลในการพล็อตกราฟ
Syntax
numpy.linspace(start, stop, num)
ความหมายของแต่ละพารามิเตอร์:
start: ค่าเริ่มต้นของอาร์เรย์ stop: ค่าสุดท้ายของอาร์เรย์ num: จำนวนข้อมูลในอาร์เรย์ (default argument = 50)
np.linspace?
# Makeup a numpy array within [-2, 2] and 5 elements
np.linspace(-2, 2, num=5)
array([-2., -1., 0., 1., 2.])
จาก -2 ถึง 2 แต่ต้องการ 9 จำนวน num=9
# Make a numpy array within [-2, 2] and 9 elements
np.linspace(-2, 2, num=9)
array([-2. , -1.5, -1. , -0.5, 0. , 0.5, 1. , 1.5, 2. ])
เราสามารถใช้ฟังก์ชั่น linspace() สร้างตัวเลข 100 จำนวนที่อยู่ในช่วงตั้งแต่ 0 ถึง \(3\pi\)
# Make a numpy array within [0, 3π] and 100 elements
x = np.linspace(0, 3*np.pi, num=100)
x
array([0. , 0.09519978, 0.19039955, 0.28559933, 0.38079911,
0.47599889, 0.57119866, 0.66639844, 0.76159822, 0.856798 ,
0.95199777, 1.04719755, 1.14239733, 1.23759711, 1.33279688,
1.42799666, 1.52319644, 1.61839622, 1.71359599, 1.80879577,
1.90399555, 1.99919533, 2.0943951 , 2.18959488, 2.28479466,
2.37999443, 2.47519421, 2.57039399, 2.66559377, 2.76079354,
2.85599332, 2.9511931 , 3.04639288, 3.14159265, 3.23679243,
3.33199221, 3.42719199, 3.52239176, 3.61759154, 3.71279132,
3.8079911 , 3.90319087, 3.99839065, 4.09359043, 4.1887902 ,
4.28398998, 4.37918976, 4.47438954, 4.56958931, 4.66478909,
4.75998887, 4.85518865, 4.95038842, 5.0455882 , 5.14078798,
5.23598776, 5.33118753, 5.42638731, 5.52158709, 5.61678687,
5.71198664, 5.80718642, 5.9023862 , 5.99758598, 6.09278575,
6.18798553, 6.28318531, 6.37838508, 6.47358486, 6.56878464,
6.66398442, 6.75918419, 6.85438397, 6.94958375, 7.04478353,
7.1399833 , 7.23518308, 7.33038286, 7.42558264, 7.52078241,
7.61598219, 7.71118197, 7.80638175, 7.90158152, 7.9967813 ,
8.09198108, 8.18718085, 8.28238063, 8.37758041, 8.47278019,
8.56797996, 8.66317974, 8.75837952, 8.8535793 , 8.94877907,
9.04397885, 9.13917863, 9.23437841, 9.32957818, 9.42477796])
เราสมารถใช้ฟังก์ชั่น sin()
หาค่า sine ของแต่ละสมาชิกในอาร์เรย์ x
แล้วกำหนดค่าที่ได้เป็นอาร์เรย์ y
# Calculate the sine of x list
y = np.sin(x)
# Plot the result
plt.plot(x, y) # Plot y versus x as lines and/or markers using default line style and color
#plt.show() # Show graph
[<matplotlib.lines.Line2D at 0x11d37a940>]
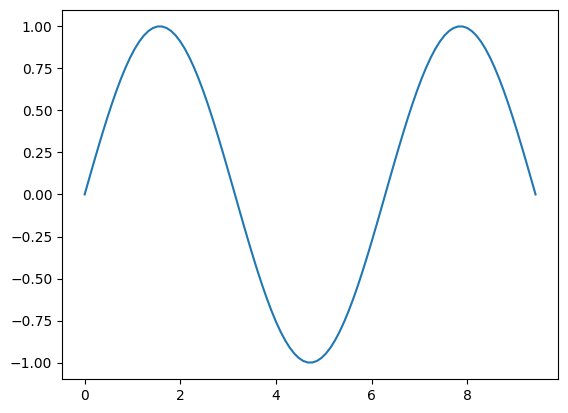
เราสามารถกำหนดสี … และอื่นๆ เองได้
ดูรายละเอียดได้ที่ matplotlib.pyplot.plot
plt.plot(x, y, 'r+') # Plot with red plusses
plt.xlabel('Angle [rad]')
plt.ylabel('sin(x)')
Text(0, 0.5, 'sin(x)')
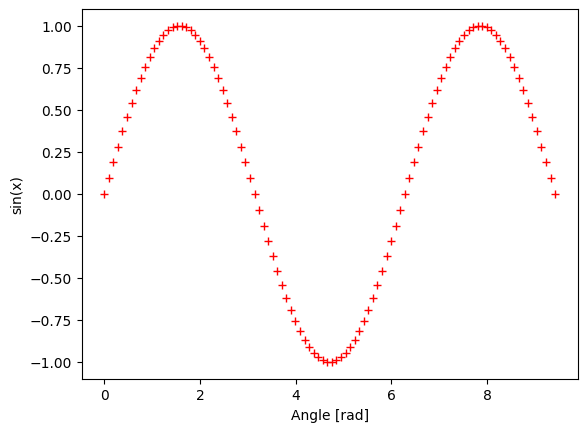
ตัวอย่างการพล็อตสัญญาณคลื่นสแควร์ (square wave) จากการรวม(ผลบวกของ) สัญญาณฮาร์โมนิกเลขคี่
สัญญาณรูปคลื่นที่ไม่ใช่สัญญาณไซน์ (Non-Sinusoidal Waveform) ที่เกิดขึ้นซ้ำๆ (มีคาบ มีความถี่ที่แน่นอน) สามารถสร้างขึ้นโดยการรวมแรงดันไฟกระแสตรง (DC) กับแรงดันคลื่นไซน์และ/หรือคลื่นโคไซน์ที่มีขนาดของสัญญาณหรือแอมพลิจูด (amplitude) และความถี่ (frequency) ที่แตกต่างกันเข้าด้วยกันได้
สัญญาณคลื่นสแควร์ (square wave) ประกอบด้วยสัญญาณรูปคลื่นไซน์ที่มีความถี่เดียวกันกับคลื่นสแควร์ (เรียกว่า สัญญาณที่ความถี่มูลฐานหรือสัญญาณฮาร์โมนิกลำดับที่-1 (Fundamental frequency or 1st order harmonic)) กับสัญญาณฮาร์โมนิกลำดับที่ 3,5 และ 7…(3rd, 5th, 7th order harmonic; เฉพาะฮาร์โมนิกเลขคี่) รวมเข้าด้วยกัน โดยที่แอมพลิจูดของฮาร์โมนิกเท่ากับ 1/N โดย N คือเลขฮาร์มอนิก (1, 3, 5, 7…)
ยกตัวอย่าง เช่น สัญญาณรูปคลื่นสแควร์ \(1V_{p-p}\) \(50Hz\) \(=\) สัญญาณรูปคลื่นไซน์ \(1V_{p-p}\) \(50Hz\) (ฮาร์มอนิกลำดับที่ 1) \(+\) สัญญาณรูปคลื่นไซน์ \(\frac{1}{3}V_{p-p}\) \(3*50Hz\) (ฮาร์มอนิกลำดับที่ 3) \(+\) สัญญาณรูปคลื่นไซน์ \(\frac{1}{5}V_{p-p}\) \(5*50Hz\) (ฮาร์มอนิกลำดับที่ 5) \(+\) สัญญาณรูปคลื่นไซน์ \(\frac{1}{7}V_{p-p}\) \(7*50Hz\) (ฮาร์มอนิกลำดับที่ 7) \(+ ...\)
ฮาร์มอนิก (Harmonic) คือ สัญญาณที่มีความถี่เป็นจำนวนเท่าของความถี่มูลฐาน (Fundamental Frequency) เช่น ความถี่มูลฐาน \(50 Hz\) ความถี่ฮาร์มอนิกลำดับที่ 3 จะเป็น \(150 (50*3)Hz\), ลำดับที่ 5 จะเป็น \(250 ((50*5))Hz\), ลำดับที่ 7 จะเป็น \(350 (50*7)Hz\) \(...\) เป็นต้น
# first, third, fifth, seventh, and ninth harmonics.
h1 = np.sin(x)
h3 = np.sin(3*x)/3
h5 = np.sin(5*x)/5
h7 = np.sin(7*x)/7
h9 = np.sin(9*x)/9
# Plot this fundamental frequency.
plt.plot(x, h1, linewidth=2) # plot with thick linewidth
[<matplotlib.lines.Line2D at 0x11d560f40>]
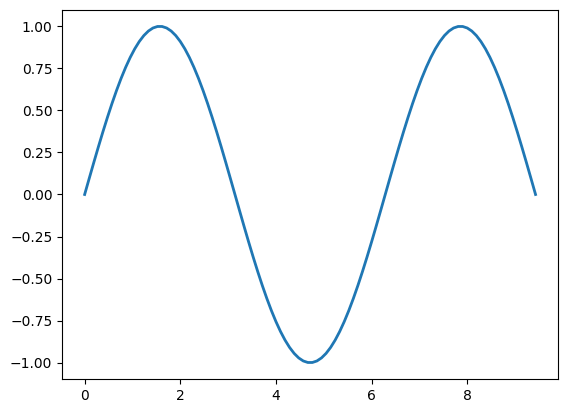
# Next add the third harmonic to the fundamental, and plot it.
plt.plot(x, h1+h3, 'b')
plt.plot()
[]
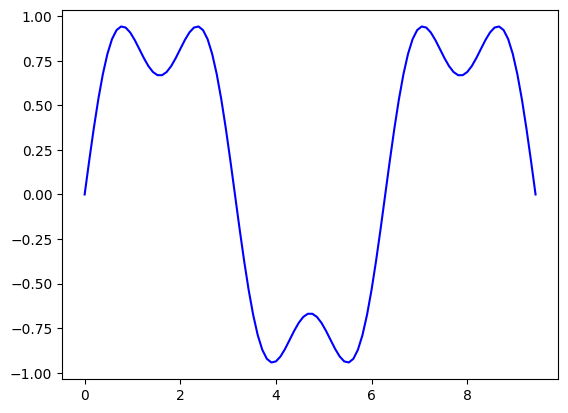
# Now use the first, third, fifth, seventh, and ninth harmonics.
plt.plot(x, h1+h3+h5+h7+h9)
[<matplotlib.lines.Line2D at 0x11d50a550>]
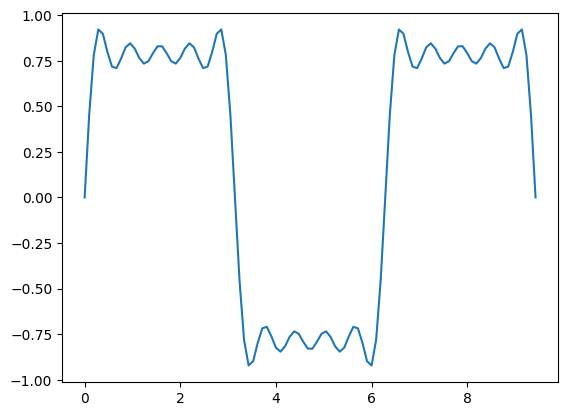
# Save graph ('eps', 'jpeg', 'jpg', 'pdf', 'png', 'ps', 'svg', 'svgz')
plt.savefig("sin.jpg")
<Figure size 640x480 with 0 Axes>
เราสามารถพล็อตหลายกราฟซ้อนกันได้
คำสั่ง plt.plot รันครั้งหนึ่งจะได้กราฟออกมาหนึ่งเส้น ถ้าหากสั่ง plt.plot ซ้ำก็จะได้เส้นกราฟออกมาอีกเส้น โดยสีของกราฟจะถูกกำหนดขึ้นโดยอัตโนมัติ ปกติแล้วเส้นแรกจะเป็นสีน้ำเงิน เส้นต่อมาเป็นสีส้ม แล้วก็สีเขียว แล้วก็เปลี่ยนเป็นสีอื่นไปอีกเรื่อยๆ
plt.plot(x, h1)
plt.plot(x, h1+h3)
plt.plot(x, h1+h3+h5)
plt.plot(x, h1+h3+h5+h7)
plt.plot(x, h1+h3+h5+h7+h9)
[<matplotlib.lines.Line2D at 0x11d34e280>]
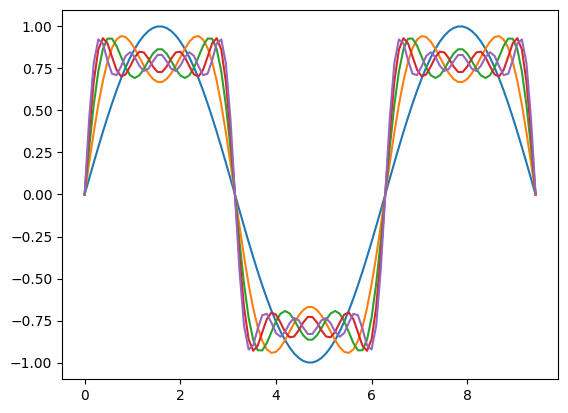
plt.plot(x, h1+h3+h5+h7+h9)
[<matplotlib.lines.Line2D at 0x11d5f6100>]
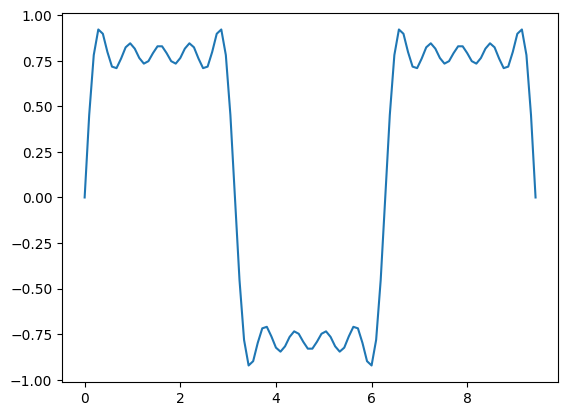
# sawtooth wave
h2 = np.sin(2*x)/2
h4 = np.sin(4*x)/4
h6 = np.sin(6*x)/6
h8 = np.sin(8*x)/8
plt.plot(x, h1)
plt.plot(x, h1+h2)
plt.plot(x, h1+h2+h3)
plt.plot(x, h1+h2+h3+h4)
plt.plot(x, h1+h2+h3+h4+h5)
plt.plot(x, h1+h2+h3+h4+h5+h6)
plt.plot(x, h1+h2+h3+h4+h5+h6+h7)
plt.plot(x, h1+h2+h3+h4+h5+h6+h7+h8)
plt.plot(x, h1+h2+h3+h4+h5+h6+h7+h8+h9)
[<matplotlib.lines.Line2D at 0x11d661cd0>]
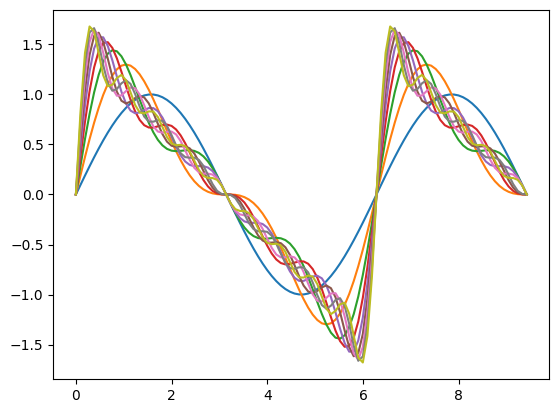
plt.plot(x, h1)
[<matplotlib.lines.Line2D at 0x11d6db730>]
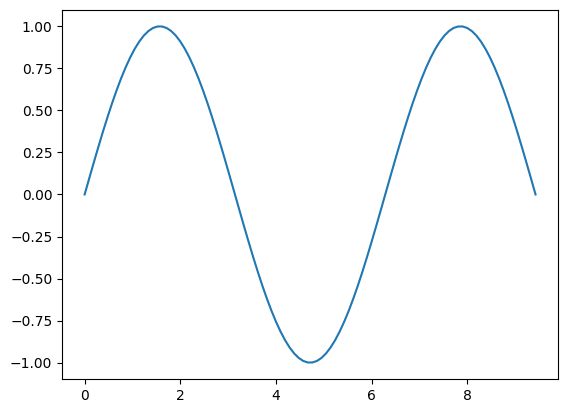
นอกจากนี้ยังมีฟังก์ชันสำหรับเปลี่ยนหน่วยที่ใช้ในตรีโกณมิติ คือ deg2rad()
หรือ radians()
ใช้สำหรับเปลี่ยนจากองศาเป็นเรเดียน และ rad2deg()
หรือ degrees()
สำหรับเปลี่ยนจากเรเดียนเป็นองศา
[Exercise]#
จากเวกเตอร์ต่อไปนี้ จงหาผลลัพธ์ของการลบเวกเตอร์ u-v จากนั้น พล็อตเวกเตอร์โดยใช้ฟังก์ชั่น
Plotvec1
u = np.array([2, 0])
v = np.array([0, 2])
# Write your code below and press Shift+Enter to execute
Click here for the solution
z = u - v
print(z)
Plotvec1(u, z, v)
จงคูณ Numpy array z ด้วย \(-\pi\)
z = np.array([2, 4])
# Write your code below and press Shift+Enter to execute
Click here for the solution
k = -np.pi * z
print(k)
กำหนดให้มีสิสต์
[1, 2, 3, 4, 5]
และ[5, 4, 3, 2, 1]
จงก๊อปปี๊ลิสต์ทั้งสองให้เป็นชนิด Numpy array จากนั้นนำไปคูณกัน
# Write your code below and press Shift+Enter to execute
Click here for the solution
a = np.array([1, 2, 3, 4, 5])
b = np.array([5, 4, 3, 2, 1])
a * b # Output: array([5, 8, 9, 8, 5])
จงเขียนโค้ดเปลี่ยนลิสต์
[-2, 2]
และ[1, 1]
ให้เป็น Numpy arraysa
และb
จากนั้น พล็อตอาร์เรย์ให้เป็นเวกเตอร์โดยใช้ฟังก์ชั่นPlotvec2
และหาผลลัพธ์ของการคูณแบบ dot product
# Write your code below and press Shift+Enter to execute
Click here for the solution
a = np.array([-2, 2])
b = np.array([1, 1])
Plotvec2(a, b)
print("The dot product is", np.dot(a,b))
จงเขียนโค้ดเปลี่ยนลิสต์
[2, 0]
และ[0, 3]
ให้เป็น Numpy arraysa
และb
จากนั้น พล็อตอาร์เรย์ให้เป็นเวกเตอร์โดยใช้ฟังก์ชั่นPlotvec2
และหาหาผลลัพธ์ dot product
# Write your code below and press Shift+Enter to execute
Click here for the solution
a = np.array([2, 0])
b = np.array([0, 3])
Plotvec2(a, b)
print("The dot product is", np.dot(a, b))
จงเขียนโค้ดเปลี่ยนลิสต์
[2, 2]
และ[0, 2]
ให้เป็น Numpy arraysa
และb
จากนั้น พล็อตอาร์เรย์ให้เป็นเวกเตอร์โดยใช้ฟังก์ชั่นPlotvec2
และหาผลลัพธ์ dot product
# Write your code below and press Shift+Enter to execute
Click here for the solution
a = np.array([2, 2])
b = np.array([0, 2])
Plotvec2(a, b)
print("The dot product is", np.dot(a, b))
เพราะเหตุใดผลลัพธ์ของการคูณแบบ dot product ของ
[-2, 2]
กับ[1, 1]
(ข้อ 4) และ dot product ของ[2, 0]
กับ[0, 3]
(ข้อ 5) จึงเป็นศูนย์ ในขณะที่ dot product ของ[2, 2]
กับ[0, 2]
(ข้อ 6) ได้ผลลัพธ์ไม่เป็นศูนย์?
# Write your answer below and press Shift+Enter to execute
Click here for the solution
เพราะเวกเตอร์ในคำถามข้อที่ 4 และ 5 ตั้งฉาก จึงได้ผลคูณดอทเป็นศูนย์
สรุปข้อแตกต่างระหว่างอาเรย์กับลิสต์#
อาเรย์จะต้องประกอบด้วยข้อมูลเพียงชนิดเดียวเท่านั้น
อาเรย์สามารถคำนวณทางคณิตศาสตร์ได้โดยตรง
อาเรย์คำนวณได้เร็วกว่า
อาเรย์มีวิธีการเข้าถึงข้อมูลภายในได้ยืดหยุ่นกว่า
อาเรย์มีคุณสมบัติการถ่ายทอดภายในชิ้นส่วนประกอบ
Change Log#
Date |
Version |
Change Description |
---|---|---|
08-08-2021 |
0.1 |
First edition |